Alexander Heinlein
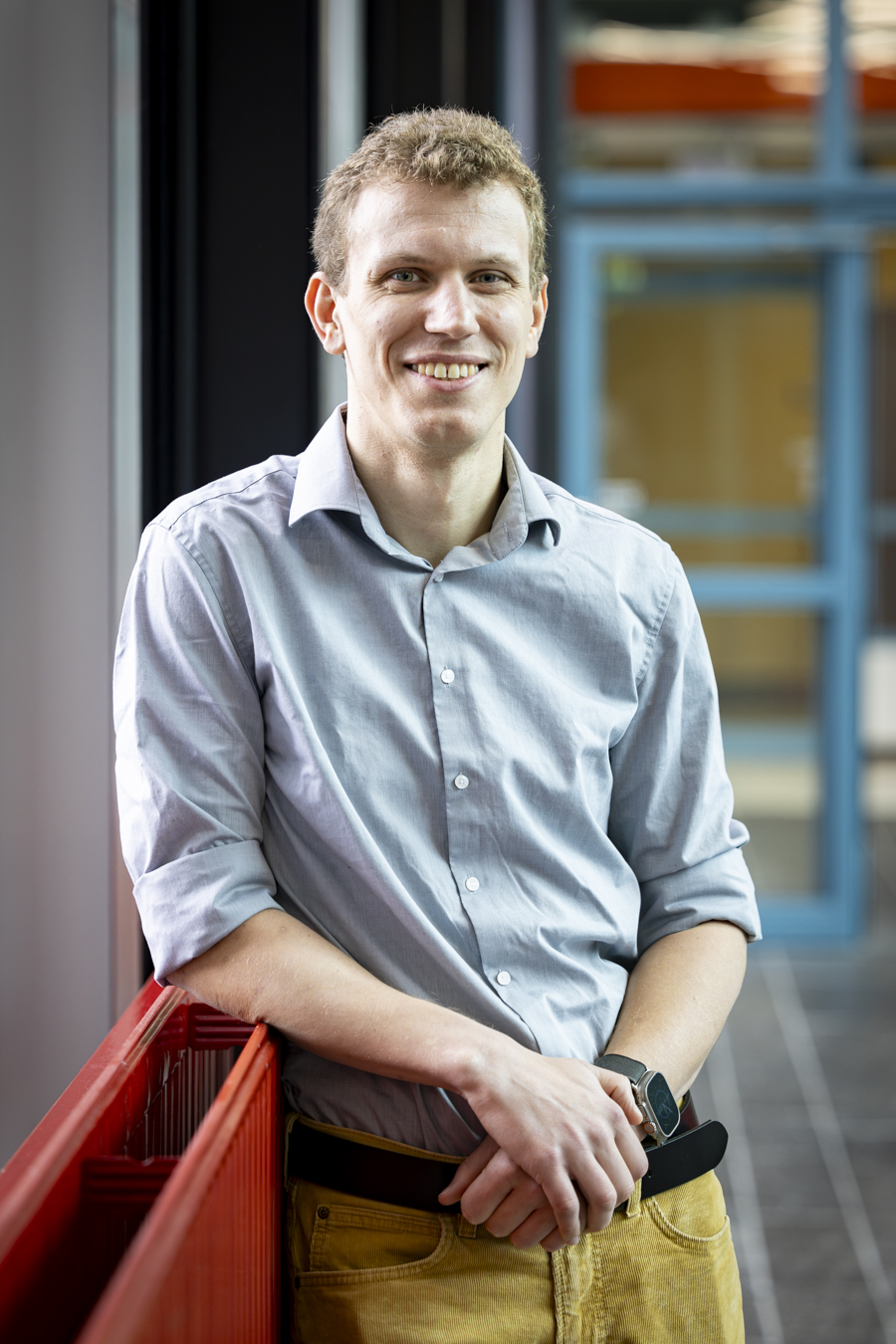
TU Delft
DIAM, Faculty of EEMCS
Numerical Analysis
Mekelweg 4, 2628 CD Delft
Room HB 03.290
Tel.: +31 (0)15 27 89135
Alexander Heinlein is assistant professor in the Numerical Analysis group of the Delft Institute of Applied Mathematics (DIAM), Faculty of Electrical Engineering, Mathematics & Computer Science (EEMCS), at the Delft University of Technology (TU Delft).
His main research areas are numerical methods for partial differential equations and scientific computing, in particular, solvers and discretizations based on domain decomposition and multiscale approaches. He is interested in high-performance computing (HPC) and solving challenging problems involving, e.g., complex geometries, highly heterogeneous coefficient functions, or the coupling of multiple physics. More recently, Alexander also started focusing on the combination of scientific computing and machine learning, a new research area also known as scientific machine learning (SciML). Generally, his work includes the development of new methods and their theoretical foundation as well as their implementation on current computer architectures (CPUs, GPUs) and application to real world problems.
news
Dec 20, 2024 | Today, Yuhuang Meng has joined our group as a PhD candidate. He will work on machine learning-enhanced numerical solvers and is co-supervised by Jing Zhao. |
---|---|
Dec 17, 2024 | On December 17, 2024, I gave a keynote presentation on Geometric Challenges in Machine Learning-Based Surrogate Models at the CASML 2024 conference at IISc Bangalore in India. The slides can be found here. |
Jul 1, 2024 | This summer, I will be visiting the Chinese University of Hong Kong to collaborate with Prof. Jun Zou. |